Digital “Twinning”: Clinical Trials Powered by AI
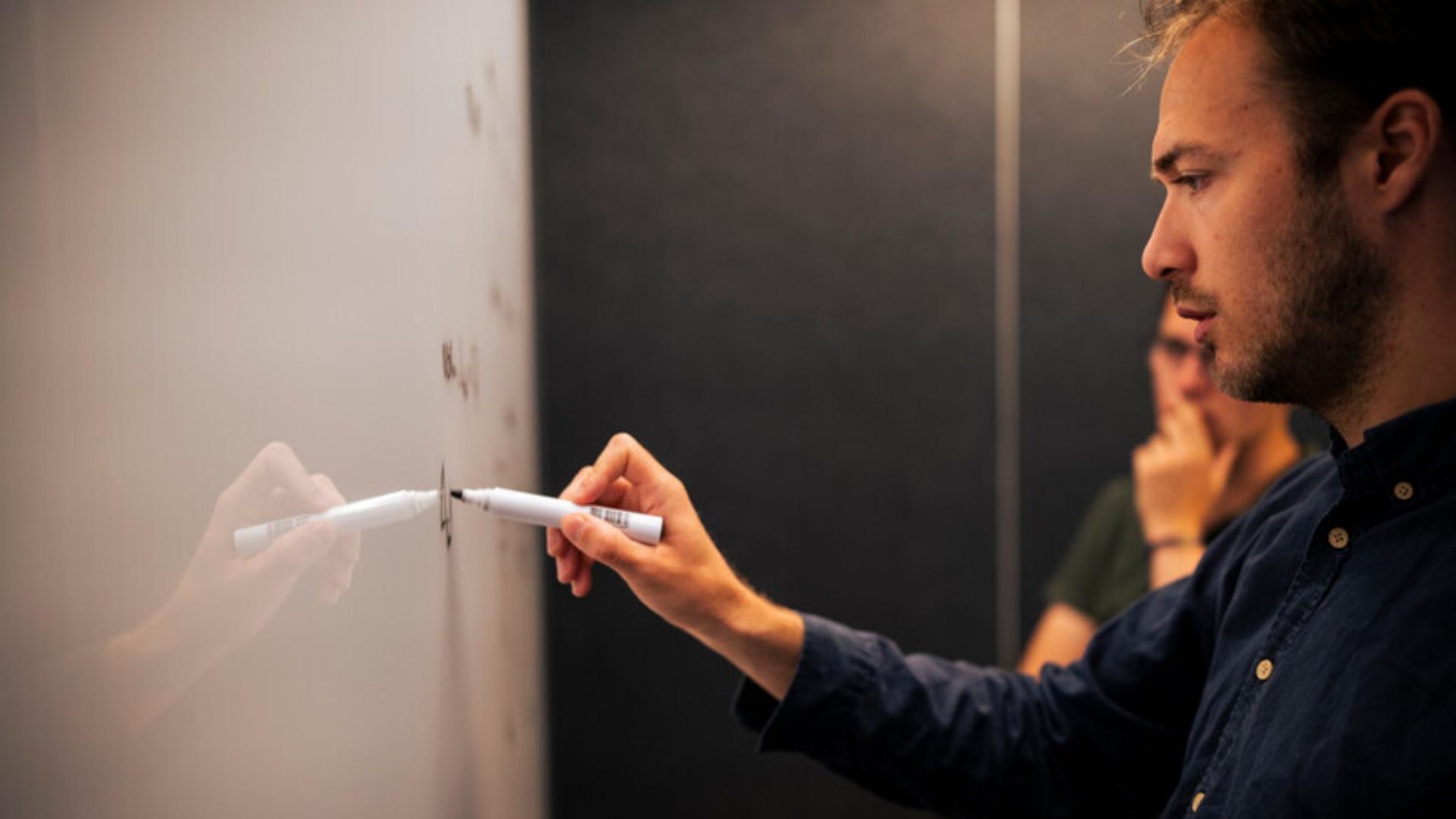
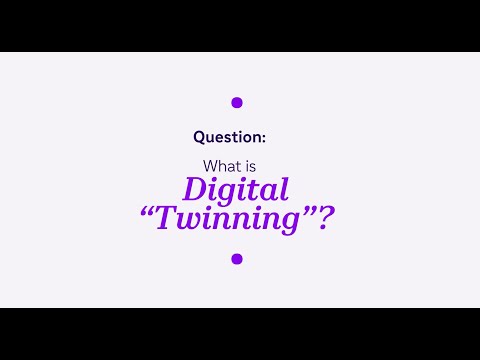
Drug development is long, resource-intensive and marked by uncertainty at every turn. Artificial intelligence (AI) has long presented itself as a potential accelerator, and unlocking its real power has been one of our greatest challenges over the last half-decade. Sanofi has led the charge, and one tangible result that we’re particularly excited about is virtual patient populations, otherwise known as “digital twins".
While real patients are still essential in late-stage studies, virtual patients allow us to test our novel drug candidates before moving them into the clinic and at different early phases during development. Through digital trials, we can conduct faster first assessments of the safety and efficacy of drug candidates with better accuracy, and potentially even reduce the number of patients needed in clinical trials.
By shifting part of the current R&D process, which may involve trial and error, to a virtual patient computer platform, we can try to improve on one of the most vexing challenges in R&D today – a 90% failure rate of new drug candidates during clinical development – and speed breakthroughs for patients
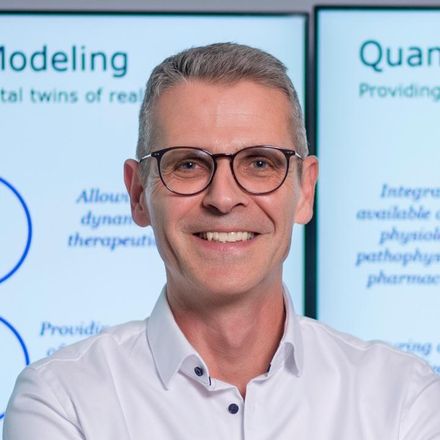
Thomas Klabunde
Global Head of Translational Disease Modelling, Sanofi
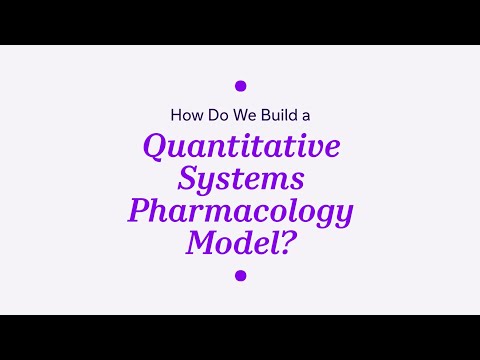
How Digital Twinning Works
Digital trials with virtual patients go beyond merely feeding patient data into an AI model. Rather, they rely on deep patient profiling and an extensive understanding of disease pathways and characteristics.
Using what is called quantitative systems pharmacology (QSP) modeling of a disease and available clinical trial data from live patients, we can create digital twins of the human patients seen in the clinic. When generating the virtual patients, we take all of the available data on disease biology, pathophysiology, and known pharmacology, and integrate it into a single computational framework.
Thomas Klabunde
Global Head of Translational Disease Modelling, Sanofi
Once this framework is in place, we can simulate an investigational compound's mechanism of action (MOA) in digital patients to understand how that compound might work on known disease pathways or drivers. We can also examine how an approved drug might perform in different treatment contexts.
Virtual trials can assess both the impact of a medicine on the disease itself and the resulting impact on clinical measures that make a difference for patients. In an asthma study, for instance, we’d examine how a compound might affect certain proteins like cytokines that control inflammation, while also evaluating its effect on lung function, such as exhalation rate and other endpoints that matter to a patient’s health, like the number of disease exacerbations within a year. This approach allows researchers to determine the potential efficacy of a compound compared to other available therapies based on their MOAs.
Virtual Asthma Patients: Test-Driving a Novel Compound
Let’s look at how this works in the real world. Our novel asthma compound showed promising data in the Phase 1b proof of mechanism trial, but it will enter a crowded landscape alongside multiple other existing treatments. Before moving it into the next clinical phase, we needed to determine if the compound could make a meaningful difference for patients over their current options. To understand this, we explored clinical efficacy in virtual asthma patients.
Our virtual asthma patients incorporated all the relevant cell types and proteins that are associated with asthma to provide a multi-scale view of the disease. To build confidence in the model, we asked it to predict the outcome of the Phase 1b clinical trial using information describing the compound, but without any information from that study. In this “blind” model prediction, the model’s results were a good match to the data observed in the Phase 1b study.
Accelerating Clinical Development with Virtual Patients
The use of virtual patients can also improve and accelerate R&D in other ways, such as:
Addressing challenges in patient recruitment
The Next Frontier
Our investment over the last several years has already generated virtual patient populations for multiple therapeutic indications. We’ve built AI programs to slash R&D times through improved predictive modelling. As a result, our teams can scale and accelerate research processes from a matter of weeks to just hours and improve potential target identification in therapeutic areas like immunology, oncology, and neurology.
Yet, there is still more work to be done. Because digital patient populations rely on existing data to generate results, current models are strongest when dealing with diseases that are caused by single gene mutations and where we have a thorough understanding of the underlying biology. In diseases such as cancer, where there are unknowns about the role of genetics and biomarkers and the tumor microenvironment has a significant impact, the scope for virtual patient populations is much smaller.
The next frontier is to build algorithms that can improve deep learning and anticipate the unknowns in more complex diseases, so that virtual patients can help improve drug development for even more conditions. Our goal is to eventually build a “biology foundation model” trained on data from biology, medicine, real-world evidence and clinical trials that can be applied widely across drug development, including predicting clinical efficacy. A project in collaboration with the BioMedX institute in Heidelberg, Germany has already begun this research.
“As we press forward in merging intricate profiles gathered from human trials, the realization of this vision becomes ever more tangible," Thomas envisioned. "Our ultimate goal is to accelerate the delivery of cutting-edge medicines to patients, ushering in a future where healthcare advancements unfold swiftly and seamlessly."
Explore More
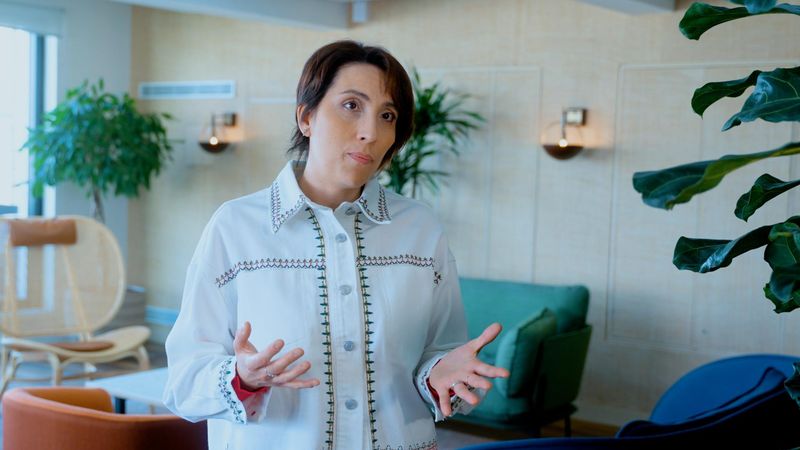
All in on AI, Accountable to Outcomes
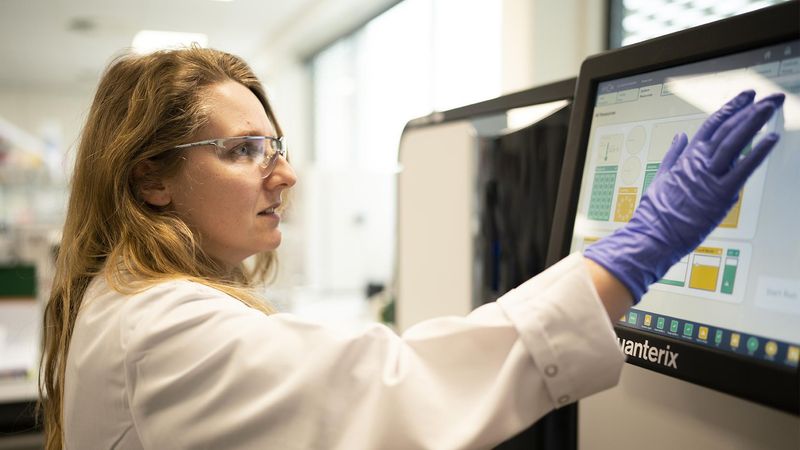
Exploring Our Digital Transformation: Strategies and Innovations
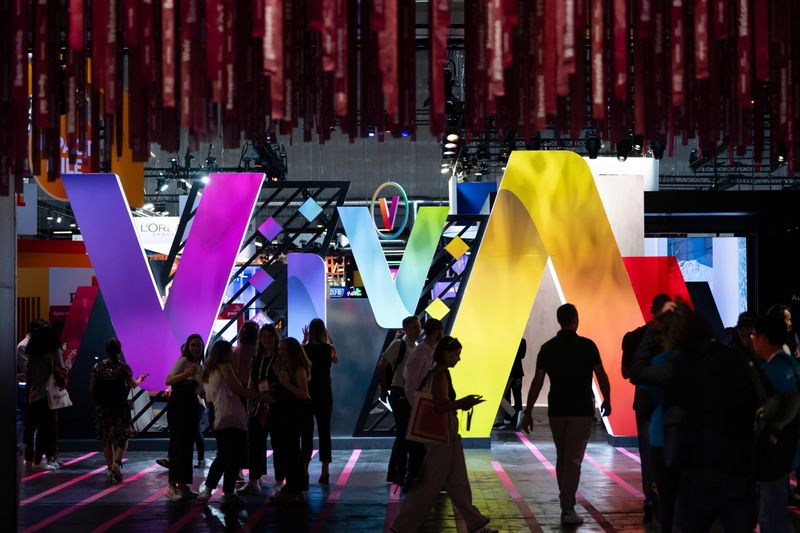