Digital and Data Science
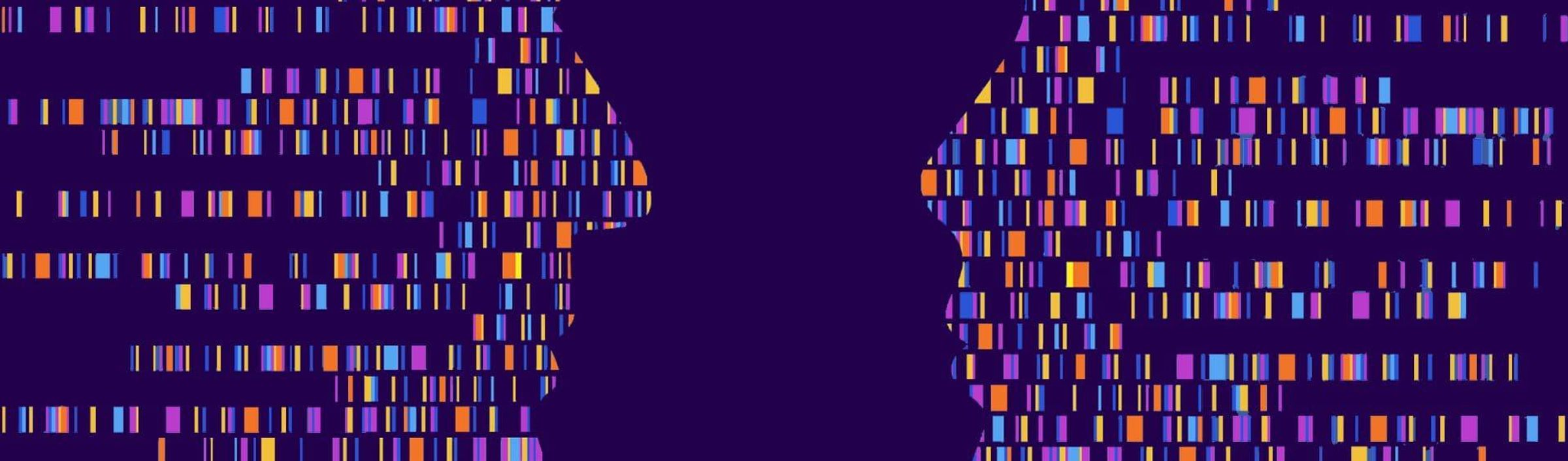
We believe data science can transform how we discover, develop, and deliver therapies. It can also help us provide better care at a reduced cost and improve outcomes by empowering people to manage their disease better.
Our data science community spans every therapeutic area at Sanofi. Working with our colleagues and partners from bench to boardroom, we’re building scalable platforms to help shorten the diagnostic journey and improve both public health and the standard of care for diseases. Our R&D focus is on specialty care including cancer, inflammatory conditions, rare blood disorders, rare diseases, vaccines and neurological disorders.
Machine learning to enable data-driven drug and vaccine discovery
We’re developing, extending, and using state-of-the-art machine learning and AI methods so we can make the best use of data in all stages of drug and vaccine discovery. We’re leveraging:
- deep neural networks and other supervised and unsupervised technologies for target identification,
- active learning for drug and molecule design, and
- graphical models for integrating clinical and molecular data to improve clinical trials.
Taken together, these approaches are transforming the drug discovery and testing process, making it more efficient, faster, and more accurate. That’s how we can best support the patients we serve.
Clinical trials and real-world evidence (RWE)
Our R&D teams are collaborating across disciplines to discover and validate digital biomarkers of disease. We use AI, bioinformatics, and other methods to find meaningful patterns in complex, multi-dimensional datasets, for example combining data from smart devices (e.g. internet-enabled spirometers), wearables, and “invisibles” used in decentralized clinical trials with electronic health records, genomics, proteomics, and real-time environmental measures.
Open science and collaboration
Open science and collaboration are helping us bring new solutions to patients more quickly. In Open Targets, for example, we’re working with academic and other industry partners to systematically improve the identification and prioritization of drug targets. Our partnerships with innovative small- and medium-sized enterprises extend our capacity to identify targets, build robust disease models based on large datasets while preserving privacy, and mine rich RWE datasets to gain new insights into biological mechanisms that drive specific diseases.
Explore more
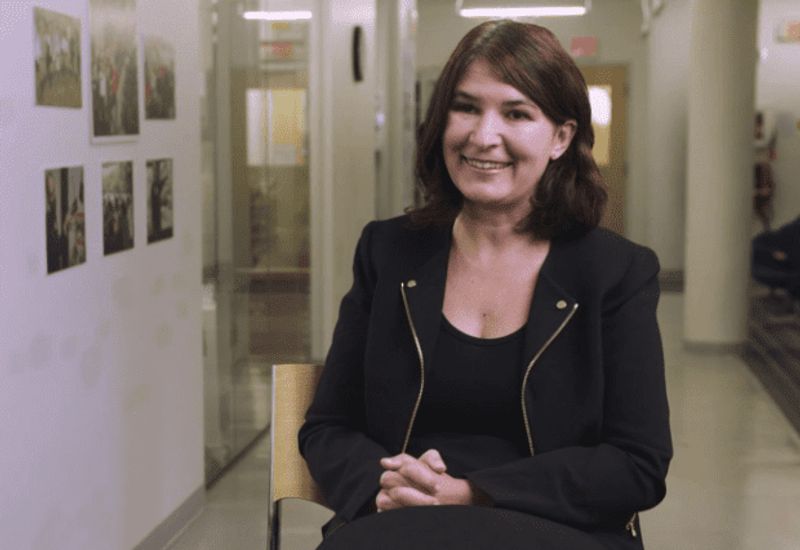
Voices from the Lab: Meet Virginia Savova
References
Many of our recent methods have been published in leading computational venues.
- Barozet A, Molloy K, Vaisset M, Siméon T, Cortés J (2020) A reinforcement-learning-based approach to enhance exhaustive protein loop sampling. Bioinformatics 36:1099-1106; doi: 10.1093/bioinformatics/btz684
- Theilhaber J, Chiron M, Dreymann J, Bergstrom D, Pollard J (2020) Construction and optimization of gene expression signatures for prediction of survival in two-arm clinical trials. BMC Bioinformatics 21:333; doi: 10.1186/s12859-020-03655-7
- Zhang W, Wei Y, Zhang D, Xu EY (2020) ZIAQ: a quantile regression method for differential expression analysis of single-cell RNA-seq data. Bioinformatics 36:3124-3130; doi: 10.1093/bioinformatics/btaa098
- Chatelain C, Durand G, Thuillier V, Augé F (2018) Performance of epistasis detection methods in semi-simulated GWAS. BMC Bioinformatics 19:231; doi: 10.1186/s12859-018-2229-8